What do we mean by AI?
The term AI is used a lot. From the context, you’ve probably guessed we’re not talking about Avian Influenza or Artificial Insemination, but instead we’re in the realms of Artificial Intelligence. But even then, what do we mean by AI? It’s something of an umbrella term now, but the phrase “artificial intelligence” was coined in 1956, to describe computers doing tasks in a way that mimics human intelligence. What tends to be referred to in current debates around AI is “Generative AI”, meaning AI designed to generate new outputs based on existing outputs.
Generative AI is by far the more contentious. More traditional AI has long been a staple of assistive software and accessibility features – it’s what powers spell check tools and voice recognition to enable dictation. However, these tend to be features within a program and aren’t generating content. Spotting typos in your essay is very different from writing passages of it; transcribing your dictation is different from composing it for you. It helps you produce your work, it doesn’t create content for you. With generative AI, the clue is in the name – it generates content. Rather than mediating the process for you, it generates material – be that image, text, music or code. AI features have been transformative for digital working for many disabled people, but as generative AI is introduced more widely, there are also potential risks. It’s the addition of these generative AI features into assistive software and their marketing as accessibility tools that this post will consider.
General concern around AI tools and features
In this post, I’m approaching AI from the perspective of assistive software and accessibility (and this is just a blog post, this is by no means comprehensive) but there are wider concerns about the use of generative AI that I need to acknowledge – each of which has an impact on use regarding accessibility. The first much discussed and yet to be tackled problem of generative AI is environmental cost. These are energy hungry tools and their water consumption alone has been cause for concern, as the data centres that power them require vast quantities of water for cooling. There are potentially beneficial uses of generative AI, but the environmental cost of using these tools means we need to be sure that they are really adding value. If there isn’t a clear use case, why is an AI element being added? These products come at significant energy and water consumption cost – their output needs to be worth this price. Disabled people are disproportionately impacted by environmental disasters and climate change. In trying to find a quick solution to a tech problem, even to improve accessibility, use of these tools could be contributing to the long term and global difficulties that increasing climate change brings to disabled people (as well as to the planet as a whole).
The second of the elephants in the server room is security. Because of the proprietary nature of algorithms, companies are not very transparent about what their tools do with data, with key security questions for any digital tool being what data is accessed, what data is stored and where it is stored. Whilst extensions can provide additional accessibility features, these constitute third party processing. If the only way to make a package accessible is to use third party tools, this potentially puts the disabled user at a digital disadvantage, potentially left with the dilemma of choosing between an accessible feature they need or keeping their data secure.
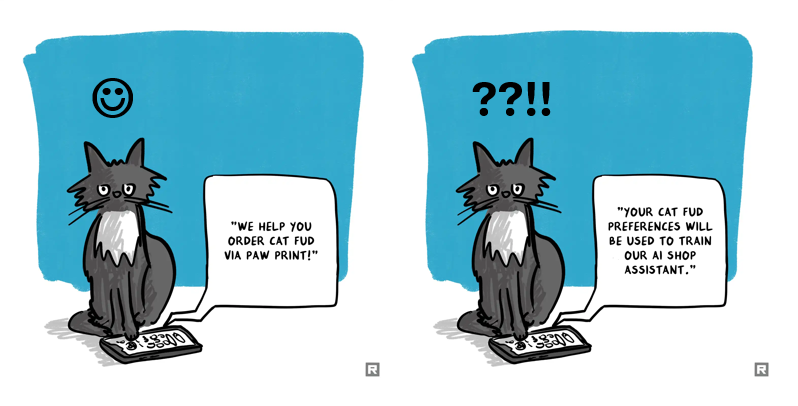
The third issue is copyright. It’s possible to use some tools without the materials being processed being used to train the underlying AI, but this isn’t standard. Many AI tools access, use and repackage data from copyrighted sources. This is a risk both with any research data or original work being used with AI tools, and also in using AI tools to access published literature. It’s a complex landscape, although this JISC introduction offers a useful overview of the key issues. From an accessibility perspective, one of the most heavily marketed academic uses of AI is for summarising articles, commonly promoted for those with SpLDs, neurodiverse individuals and anyone with cognitive load issues. But where have you sourced the article from? If it’s from an academic library subscription, can you use it in this way? Many academic publishers have clauses in agreements specifically prohibiting use of AI tools with their published materials – covering articles, books and datasets. In which case – why pay for a tool that promises to summarise material for you, when you can’t use it without breaking the law or specific user agreements? The legal situation is still unclear, but historically, publishers are litigious – time will tell how this will pan out. But aside from the legal questions are the ethical ones – has the author agreed to this use of their work? Does using a summary tool inherently mean offering up an author’s intellectual property to train AI? It’s a fast moving and still developing field. We’re still trying to assess what long term impacts might be and where problems have arisen, few (if any) legal precedents exist. Hopefully some of these problems will be resolved and there is movement towards this – developers have created models which only work with open data, so tools which work within copyright law are available. However, until this is the norm for the products offered from the big tech companies, this remains problematic. Encouraging users to build working practice that depends on these tools risks offering an assistive tool, only for the legal issues to see it being withdrawn, or offers a potentially assistive tool that supports one group at the expense of another – supporting those disabled users who find it beneficial by exploiting the intellectual property rights of the authors. Both the security and copyright concerns put disabled users in a very awkward position. By using generative AI unchecked in products, the digital tools which could offer transformative support put them at risk – of poorly protected data, or breaking GDPR or copyright law, or exploiting the intellectual property of others.
Within an assistive software and accessibility context
There is also the risk of these users falling for promises which these generative AI tools simply can’t fulfil. Advertising overpromising new features isn’t new and AI features aren’t alone in being offered as solutions to complex problems. Snake oil is nothing new and products promising to solve the seemingly insoluble have been around for most of recorded human history. These products find a market because people have problems they desperately want help with and the sales pitch promises a solution. Some generative AI packages have been promoted towards those with ADHD, SpLDs and any cognitive processing conditions as tools which will revolutionise their productivity and workflow. But these promises are typically unproven – in many cases the software and tools are simply too new to have any properly demonstrable benefit to any specific demographic. Even with assistive software known to benefit disabled people, these don’t present uniform solutions to accessibility issues. Different individuals with the same condition will experience these differently, some may find it advantageous, others may find it of little help. As such, the wide ranging benefits promised by these tools should be understood as marketing, with anecdotal support at best. The ability for many people to safely use these at work is also sidestepped in marketing campaigns. The significant issues with copyright and security mentioned above mean that in many workplaces, these tools won’t meet cybersecurity and GDPR requirements. Even where there is no workplace barrier to using these, the user is at risk of effectively being used as training data as a disabled user. There are ethical questions about the marketing of tools specifically to these user groups (many of these are costly subscriptions) and the lack of security they offer, as this can be seen as exploitation of a potentially vulnerable group. Stability is also an issue – encouraging people to rely on tools which are subject to change and price hikes risk people coming to depend on tools which may be significantly changed, withdrawn or suddenly unaffordable to them. The cost of subscriptions to these tools can in itself be prohibitive.
These products find a market because people have problems they desperately want help with and the sales pitch promises a solution.
It’s not always clear what the addition of generative AI brings to a product, other than reflecting current trends and potentially adding another element to a sales pitch. For example, generative AI elements are being introduced to academic search tools, promising to find you better or more helpful results. However, the level of description and detail required in a prompt that would return useful results is equivalent to the effort and similar in construction to a structured search – it is no less effort, and the search parameters of the tool are less clear. So what value is this adding? Are consumers being charged more for new features which add little (or nothing) to a product?
Going further – could the addition of generative AI features actually make a tool less reliable? One of the most promoted areas of generative AI within an accessibility context in higher education (although also more widely) is the ability of Large Language Models (LLMs) to summarise longer documents. In terms of academic articles, this purpose is already served, at least in part, by the abstract, which typically acts as a summary of the intentions of the authors and their research. Whilst the abstract is inevitably subject to the bias of the authors, this is arguably already serving the purpose of a summary. A known issue in the use of LLMs is accuracy. These can often generate false answers, sometimes termed “hallucinations”. This is a bit of a misnomer. The LLM doesn’t think, it is designed to present the user with something that looks like other answers in the training data. So it will present something modelled on other answers – even if those answers aren’t relevant. Journalists and others have assessed the accuracy of various LLMs in various ways – this article about asking basic historical questions demonstrates that the answers generated can be extremely unreliable, even on the topic of easily verifiable facts. As such, can these tools be relied upon to accurately summarise an article? This might be fine if the summaries are just being used to identify potentially useful articles, which the user then reads in full and draws their own conclusions, but is more problematic if the user intends these summaries to form the basis of a literature review. Even if just for an initial review of documents, an LLM may be worse at summarising than you might expect. This review of LLM efforts to summarise reports suggests that instead of summarising documents, these tools actually shorten them. That is to say they represent a few key sentences from the source, but do not provide any actual overview of the work and can fail to include important elements of the work in the generated summary. Uncritically presenting these tools as assistive aids to disabled students and academics is to put them in a position of undermining the accuracy of their work.
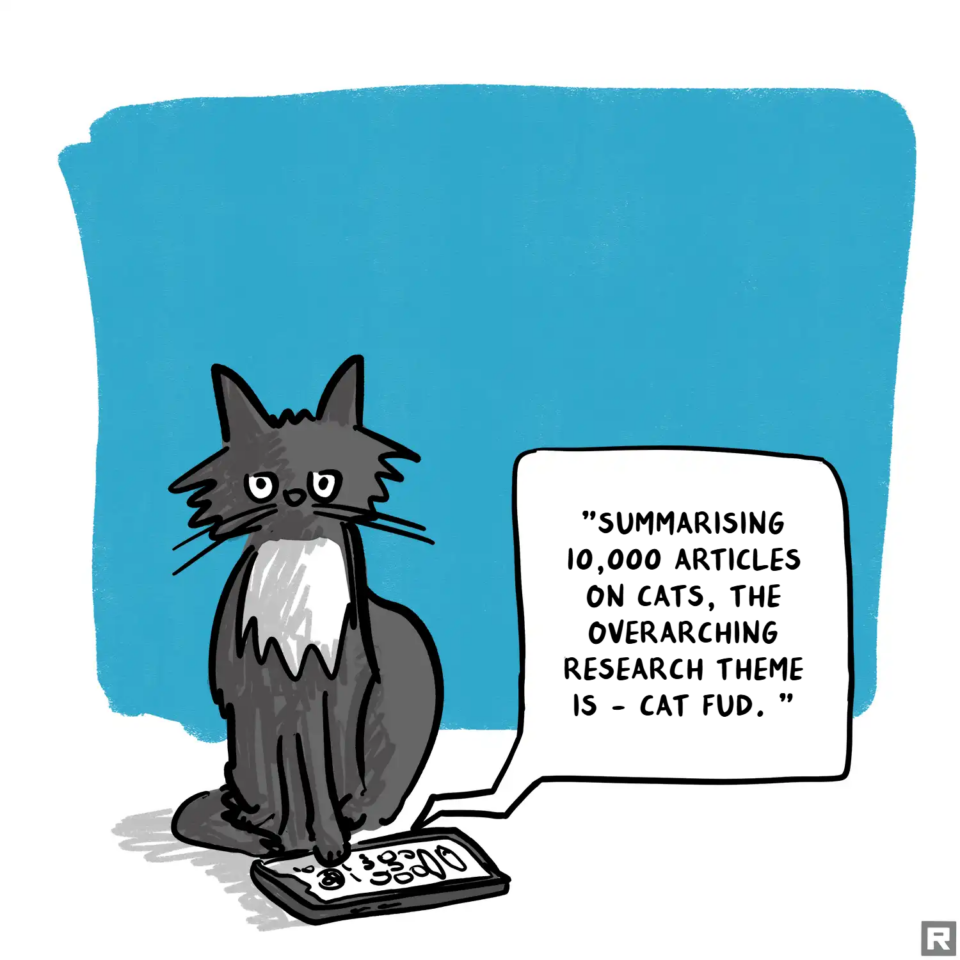
This isn’t the only area where accuracy in reflecting original sources is known to be problematic. Without further development the use of generative AI could also perpetuate inequalities by failing to acknowledge or address biases in underlying training data. Generative AI tools learn from vast data sets, often from across the internet. And whilst the internet hosts all sorts of information, it’s also home to a lot of misinformation and disinformation. Disturbingly, analytical AI tools have been shown to replicate social biases, including against disabled people, with the potential for real world harm. In creating an answer that looks like other answers, LLMs will replicate biases. Both text and image Generative AI outputs have been shown to recreate stereotypes. False information or data is problematic but may be easier to spot than more insidious replications of bias. Whilst issues around racial biases and misogyny have received more public attention, ableism in AI is also an issue. With AI training content based on biased data and materials containing ableist attitudes, AI output will reflect and perpetuate these issues. Although often marketed to groups of disabled people as assistive, generative AI tools have the capability to perpetuate stereotypes and do harm to disabled people.
Is generative AI an answer or avoiding the issue?
Accessibility by design is always easier than a retrofit, whether addressing physical or digital accessibility. Universal design that accommodates different needs from the start is a far more inclusive approach, anticipating a diverse audience rather than addressing different requirements as an afterthought. Currently, the way in which some generative AI tools are marketed buys into this perception of accessibility as an additional task, rather than an approach to take throughout your work. These tools are offered as ways to render your content more accessible without you having to think about it – you don’t need to take a more inclusive approach, the tool will resolve the problem of accessibility for you.
An example of this is auto generated alternative text – using either inbuilt platform tools or external tools to generate you a short image description, rather than composing the text yourself. So if you haven’t written alt text before, seeing the generated text can be a helpful starting point – how does the software interpret the image? But the skill of image description is providing the nuance of context. If the image isn’t just decorative, why has it been chosen? Did you add it as a typical example, or because it is an exception? What is it about that particular picture that prompted you to choose it to support your message? Essentially your image description needs to impart the information that a sighted user would get from the image that a user with no sight would not – and the auto generated text won’t know what it was you wanted to convey. The automatically generated text can provide a starting point, a description you can edit or add to as needed, but to use that text as your default won’t necessarily serve the purpose of the image description. Default use without checking or editing a generated description perpetuates inequality. By providing an alternative text your content appears accessible, but unless it is meaningful, this just gives the illusion of inclusivity without actually providing it.
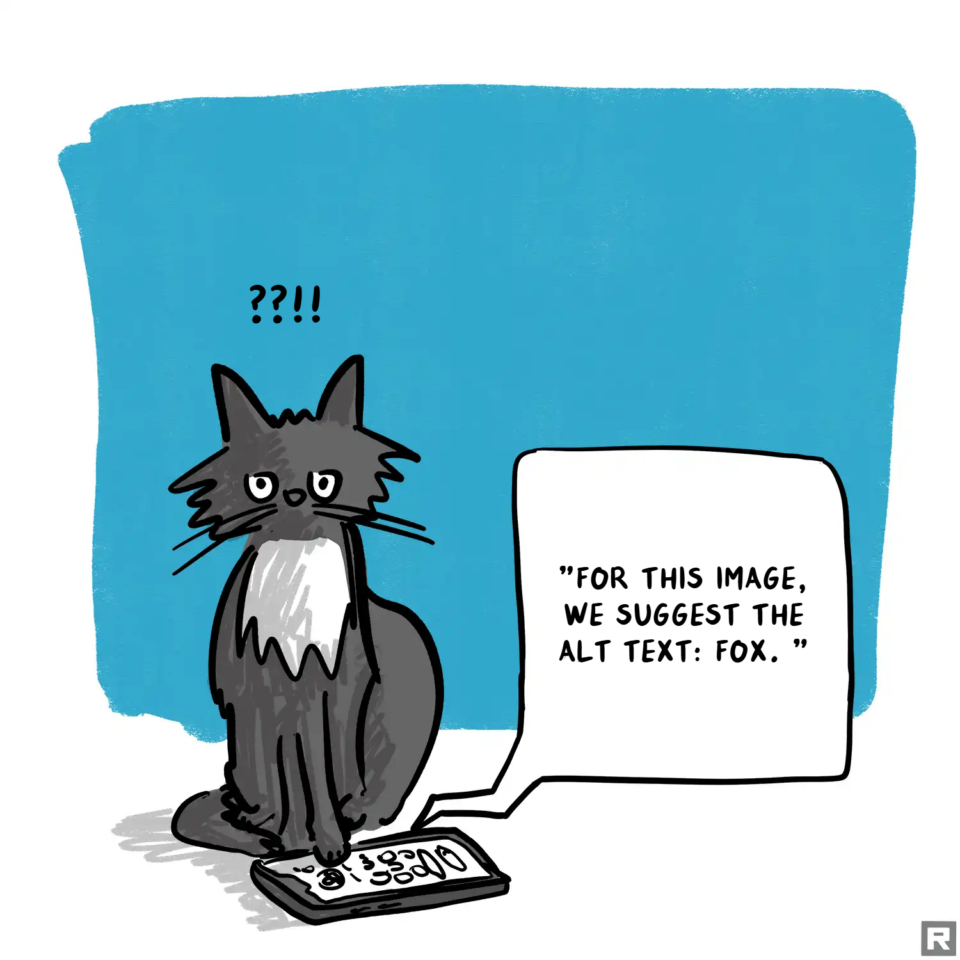
Accessibility checkers are another area of automation. I’m a big fan of Microsoft Office’s inbuilt accessibility checker tools, of the Grackle extension for Google workspace and BlackBoard Ally for the BlackBoard VLE. These highlight potential problems and offer suggestions to resolve these. But the important part is the review – these don’t correct issues for you but show potential problems and it is for you as the user to make the necessary changes. These tools support accessible practice – they act as reminders and spot mistakes, but the user still needs to review their work. Accessibility checker tools also aid development of web tools. These run automated checks to identify accessibility issues, but like AI generated image descriptions, these tools need to be a starting point. They can help in the creation of a tool that is technically accessible, but they cannot replace work with disabled users to make a tool practically usable. For example, automated accessibility checkers can help you determine if a webpage can be accessed with a screen reader, but it cannot tell you about the experience of someone using a screen reader navigating a site. Automated accessibility checkers help clear the first hurdles of making resources digitally accessible, but they can’t replace user research and user testing as a means of understanding user experience. However, AI tools are being marketed as a better means of checking web accessibility, with claims that “AI algorithms can analyze user behavior data to identify common navigation issues faced by users with disabilities”. Disabled users find their own workarounds and are frequently highly skilled users of assistive technology in navigating resources. What user behaviour data is being analysed and where is it from? Without direct input from disabled people, can this truly reflect experience? These tools have great potential to support accessible development, but they can’t replace final rounds of user testing by disabled people to determine how truly accessible an online resource actually is. Otherwise we enter a new automated era of disability erasure. The disability rights campaign slogan has long been “nothing about us without us“. Historically this has been the design of policies, services and products for disabled people by people with no disabilities – we could now risk the design process excluding disabled people in favour of AI automation.
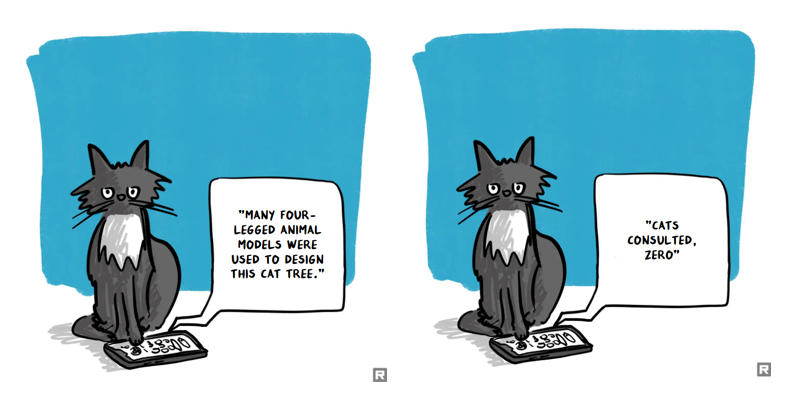
Away from academia, a pop culture example of this is found in the Marvel miniseries Echo. Maya, the series’ protagonist, is deaf and communicates primarily through American Sign Language (ASL). As the protege of Wilson Fisk (also known as Kingpin), their conversations are mediated by an ASL translator, with Fisk only learning a few key phrases. Later, Fisk gives Maya an augmented reality contact lens, which superimposes ASL signing over him when he speaks, removing the need for a translator. Maya becomes angry – despite saying she is like a daughter to him, he will not undertake to learn ASL to communicate directly with her. This example encapsulates the discomfort disabled people can feel in seeing those around them relying on tech for inclusivity, rather than changing their own behaviour. It illustrates the attitude that direct engagement with disabled people – in this case provision of a human translator or learning to communicate directly – can be outsourced to tech, that’s it not something worth spending time on. The feeling of being excluded, of seeing inclusion being an afterthought or seen as an active nuisance, is something with which many disabled people are unfortunately very familiar.
This promotion of AI tools as a convenient way of making content supposedly more accessible with no effort raises the question of why can’t we prioritise building accessibility features rather than outsourcing it as an afterthought? Accessibility shouldn’t be relegated to automation to tackle annoyances – universal design is a more inclusive approach, not relying on AI tools to fix problems manufacturers cannot be bothered to address. It can also be seen as an oversimplification of situations, with reliance on automated or AI tools eliminating nuance and giving a poorer experience for disabled users.
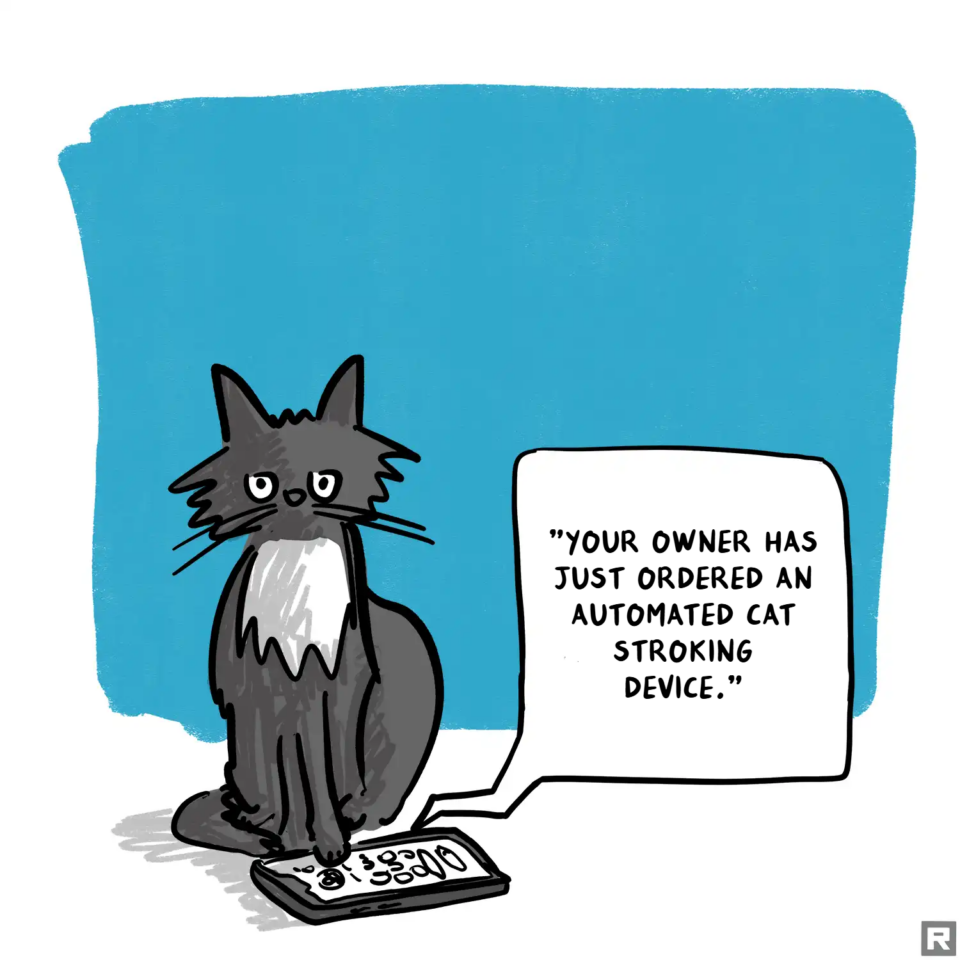
So in conclusion…
Do I have a definitive conclusion about generative AI in assistive software or generative AI tools presented as accessibility supports? I’m not sure that I do, but this is a blog post and not a thesis, so maybe that’s alright. Some people may think this is a highly critical post – perhaps in some ways it is. And full disclosure – I’m a librarian by training, I work in digital accessibility and I’m disabled. I will readily admit that all of these factors have shaped my views on the subject. I’m not here to inherently attack the concept of generative AI, but I wanted to redress material I have seen uncritically promoting these tools, particularly the marketing to disabled consumers. Traditional AI features have been transformative for disabled people, and I hope that there are generative AI tools which will have the potential to be so. But I don’t think I have seen one yet. What I have seen is the promotion of tools with questionable security and copyright compliance to groups in search of support, encouraging disabled people to use and subscribe to tools which could make them vulnerable to cybersecurity issues or be used to call their work into question. For me, there is clearly potential in this field to better provide digital support, but there are at least as many unanswered questions – and I’d want to see more of these addressed before promoting adoption of these tools. Most of all though, I feel discomfort with the way that some of these new tools are perpetuating the perception of accessibility as an afterthought, promising to automate accessible features without actually providing nuance or attention to the experience of disabled users reliant on these outputs to engage with content. However generative AI ends up being used, I believe more inclusive design is necessary, building in accessibility to products and services throughout their design, development and implementation. So how do I feel about generative AI and digital accessibility for now? I’d recommend anybody to ask critical questions of claims made for these tools; to think about what you’re automating and why; and whether and however you choose to use generative AI, to adopt an inclusive approach and not regard accessibility as an afterthought.
Cartoons created by Lilian Joy using remixer.visualthinkery.com.
“The LLM doesn’t think, it is designed to present the user with something that looks like other answers in the training data.” is probably the best shortest correct and understandable definition I have seen so far.